The multi-trillion dollar oil and gas industry has historically been characterized by long megacycles of shifting supply and demand, with various shocks along the way.
From 1990 to 2005, total returns to shareholders (TRS) in all segments of the industry, except refining and marketing companies, exceeded the TRS of the S&P 500 index. This was a period of strong demand growth and stable prices, thanks in part to OPEC's efforts to maintain price discipline.
However, from 2005 to January 2020, the industry failed to keep pace with the broader market. Despite strong demand and effective supply, the average total returns to shareholders (TRS) growth of the oil and gas industry was about seven percentage points lower than the S&P 500.
In recent years, the rise of shale has made it more difficult for OPEC to maintain market share and price discipline. While OPEC cut production by 5.2 million barrels per day (bpd) from 2016 to 2020, shale added 7.7 million bpd over the same time period, limiting price increases. The rapid development of new resources has also made it difficult to repeat the run-up in prices that occurred from 2000 to 2014, as the industry can now turn on ample supply in a matter of months rather than needing a decade to find and develop new resources.
Historically, price wars wipe out poor performers and lead to consolidation. But the capital markets were generous with the oil industry in 2009–10 and again in 2014–16. Many investors focused on volume growth funded by debt, rather than operating cash flows and capital discipline, in the belief that prices would continue to rise and an implied “OPEC put” set a floor.
Looking ahead to the post-COVID-19 era, the industry faces intense competition, technology-driven rapid supply response, flat to declining demand, investor skepticism, and increasing pressure from the public and government on climate and environmental issues. In order to thrive in this new environment, companies will need to embrace bold structural moves, innovation, and profitable operations in tough conditions. Those that are able to reposition their portfolios and transform their operating models will be the winners
It is common for large enterprises in the oil and gas industry, such as Exxon Mobil, Shell, and Total, to use technology, including artificial intelligence and data analytics, to gather and analyze data in order to optimize their operations and improve efficiency. This can include tracking inventory, optimizing production and distribution processes, and identifying areas for cost savings. By leveraging these tools, companies can better manage their cashflow and make more informed business decisions.
What's the value of doing so, let's look at 1 example.
Errors in payments for oil barrels can occur for a variety of reasons, such as incorrect measurements, misunderstandings about the terms of the contract, or discrepancies in the quality of the oil being delivered. These errors can lead to lower payments for producers, which can have a significant impact on their bottom line, especially if they are producing large quantities of oil.
To ensure that they are being properly compensated, producers need to analyze a large amount of data in order to present defensible data to their buyers. This can be a costly and time-consuming process, especially for producers who are making 400,000 barrels of oil per day and need to analyze over 240 million data points in a month. In order to efficiently handle this volume of data, many large operators have implemented large control rooms and hired IT staff to leverage data analytics and other tools.
However, with the recent advancements in AI, even smaller operators who are producing as little as 10,000 barrels of oil per day can use AI and machine learning to analyze 8 million data points and run 112 million AI/ML computations to get the same level of analysis as a larger operator. This allows smaller producers to more effectively defend their payments and ensure that they are being fairly compensated for their oil.
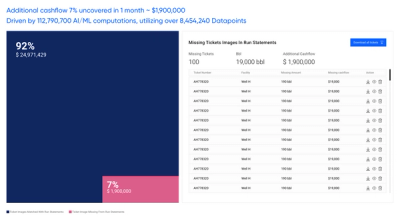
The 1st step is to estimate cashflow on a daily basis. Oil is sold from tanks, so every outflow should be accounted for
Given below are steps used by AI and machine learning or can be duplicated with manual labor with excel spreadsheets to analyze data and ensure accurate payments for oil producers. This is also enabled smaller operators to operate without the need for Truck last units
Step 1: Correct erroneous data from tank level sensors. Tank level sensors can sometimes provide inaccurate data, which can lead to errors in payment. To address this issue, an AI model can be trained to identify and correct any erroneous data from tank level sensors. This helps to ensure that the data being analyzed is accurate and reliable.
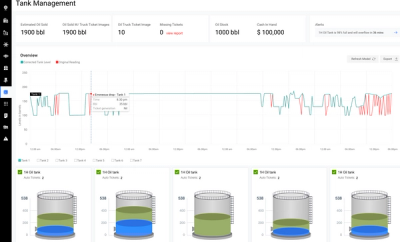
Step 2: Analyze data to estimate cash flow. Once any bad data has been removed, it can be analyzed to determine the unique signature of a truck ticket. This signature can be used to estimate the cash flow for the operator. We would use the oil price based on real time traded commodity prices to estimate revenue

Step 3: Reconcile estimated cash flow with physical truck tickets. Use AI-powered OCR to process truck tickets. Thousands of truck tickets can be uploaded at once and an AI-powered OCR (optical character recognition) system can be used to autonomously associate each ticket with the correct lease, tanks, and tank level drop signature. This can save time and reduce the risk of errors in the data entry process.
Step 4: Reconcile truck ticket numbers with payment slips. At the end of the month, the truck ticket numbers can be autonomously reconciled with the payment slips from the purchasers. Any unpaid truck tickets will be flagged, and associated images with proof of tank level drops will be made available to the operator to send to the purchaser as proof for additional payment.
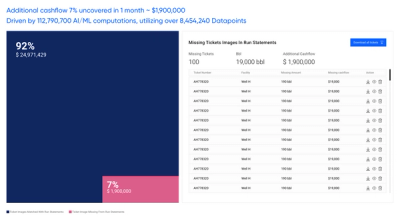
As mentioned above Sotaog's AI-powered solution can analyze over 240 million real-time data points, instantly spotting and providing defensible data to ensure Oil and Gas operators can get paid back every cent they are owed. Just like how Exxon Mobil and Shell use technology to track their inventory and sales, our platform can drive significant value for operators. Don't let errors cost impact the business - let AI handle the heavy lifting and boost your bottom line.
We offer a range of pre-built AI models that can help you to analyze and predict trends using data from real-time sensors, PDF documents, manual data, and other large datasets. Our cashflow centered solutions are easy to use, with no additional hardware or installation required and all technical consulting hours included.
With Sotaog, getting started is easy. We can take data in the format operators have it, and it typically takes just 2-3 meetings to provide you with an analysis and recommendations for driving additional cashflow.
And for a limited time, we're offering a $0 proof of concept so you can see the value of our solutions for yourself.